Cloud data warehouses have revolutionized the way organizations store, analyze, and manage their data. These powerful data platforms offer scalability, flexibility, and ease of use, allowing businesses to efficiently make data-driven decisions, run AI/ML models and build new data applications. However, when it comes to accessing and understanding the metadata associated with the data stored in these warehouses, not all providers are equal.
In this blog post, we will delve into the metadata capabilities of the leading cloud data warehouses and analyze the information they expose through their APIs. To provide you with a detailed overview, we have carefully selected five prominent cloud data warehousing solutions in the market: Amazon Redshift, Databricks, Google BigQuery, Microsoft Synapse, and Snowflake.

Under each category we’ve counted the amount of items each vendor covers. We didn’t get into the details – either it covers it (=1) or not (=0). The vendor with the highest score is considered the leader, followed by its no. 2.
Before we dive into the comparison, it’s important to note that the information collected for this analysis was gathered from the providers’ websites. While we aim for accuracy, it’s essential to acknowledge that this information is subject to change over time. Moreover, different users may prioritize categories differently based on their specific preferences. Additionally, factors such as cost, performance, user experience, integrations, and more were not covered in this analysis. Therefore, this blog post should be regarded as an overview of each vendor’s capabilities in specific domains (under the assumption that the metadata they’re exposing reflects it), and their focus on developer communities through API exposure.
Now, let’s explore the different categories of comparison, starting with the query level:
Query Level
At the query level, metadata provides valuable insights into the cost, performance, and resource utilization associated with executing queries. Let’s explore the metadata capabilities of the leading providers in this domain.
Cost:
When it comes to exposing metadata about query costs, Amazon Redshift and Google BigQuery lead the pack. These providers offer information about estimated cost, execution cost, storage cost, transfer cost, and more. Such data helps users optimize their queries and effectively manage their cloud resources.
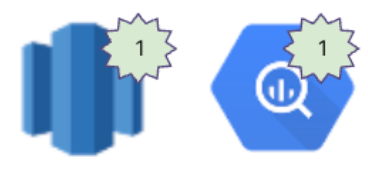
Performance:
In the performance category for query metadata, Snowflake takes the lead, closely followed by Databricks. These providers offer valuable insights into execution time, query optimization techniques, query plans, caching strategies, concurrency management, and result size estimation. Understanding these aspects enables users to fine-tune their queries and enhance performance.

Resources Consumed:
The resources category focuses on providing information about resource utilization during query execution, including CPU, disk, I/O, memory, network, and more. Amazon Redshift leads the way in this category, followed closely by Snowflake. These providers expose detailed metadata that helps users understand resource consumption patterns and optimize their workloads accordingly.
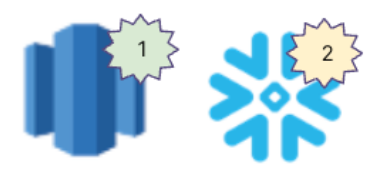
User Info:
The user info category provides insights into session details, activities, and general user information. Microsoft Synapse emerges as the leading provider in this category, followed by Databricks. These providers offer comprehensive metadata that enables effective user management and monitoring.

When considering query metadata as a whole, Snowflake emerges as the leading provider, followed by Microsoft Synapse.

Table Level
At the table level, metadata provides critical information about the structure, organization, and characteristics of the stored data. This information is crucial for optimizing queries, ensuring data governance, and enabling efficient data analysis. Let’s evaluate the leading providers in this category based on the metadata they expose.
Analytics:
The Analytics category encompasses information such as partitioning, clustering, replication, and more. Among the top providers, Amazon Redshift leads the pack, followed closely by Snowflake and Microsoft Synapse. These providers offer comprehensive metadata about partitioning strategies, key-value pairs, distribution style, sort key columns, and more. Understanding these analytics-related metadata attributes aids in efficient data organization and analysis.

Governance:
Metadata related to governance ensures that data is managed and accessed securely and efficiently. Snowflake emerges as the leader in this category, followed by Google BigQuery. These providers offer extensive information about ownership, access and roles, creation and modification details, expiration, documentation, and views’ refreshes. Such metadata empowers organizations to implement robust data governance practices.

Observability:
The observability category focuses on providing insights into data properties such as file size, row count, compression ratio, and more. Once again, Snowflake takes the lead in this category, closely followed by Google BigQuery. These providers offer comprehensive metadata that aids in understanding data distribution and storage efficiency. Leveraging this metadata, organizations can gain valuable visibility into their data assets.
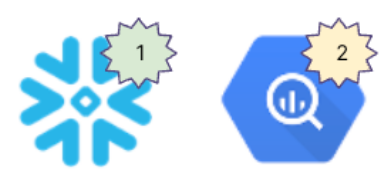
Field Level
At the field level, metadata provides information about the individual columns within the tables, including data types, lengths, encoding, and more. Let’s examine the leading providers’ capabilities in exposing metadata at this level.
Analytics:
Amazon Redshift secures the top position in the analytics category for fields metadata, followed by Databricks and Google BigQuery. These providers offer detailed information about partitioning strategies, encoding techniques, and sort-key attributes. Such metadata enables organizations to optimize their data structures for efficient analysis.
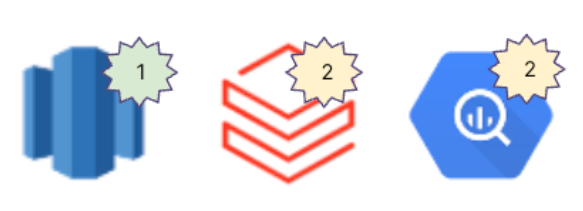
Governance:
Both Snowflake and Amazon Redshift lead the way in the governance category for field metadata. They provide valuable information such as data types, length, precision and scale, documentation, default values, access privileges, and granted roles. This metadata ensures data integrity, compliance, and secure access.
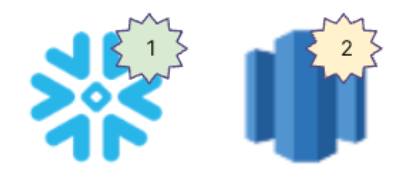
Observability:
Google BigQuery takes the lead in the observability category for field metadata. It offers valuable insights into value statistics (min, max, count, histogram, etc.), column mode (required, repeated, nullable), and more. Amazon Redshift and Databricks follow closely in this category. Utilizing this information, organizations can gain a deeper understanding of their data distribution and quality.
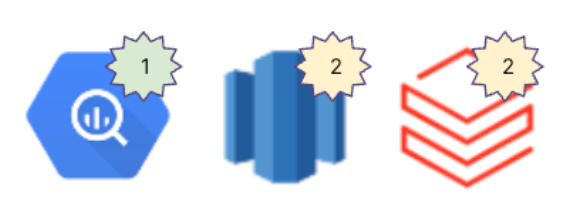
In terms of field metadata, Amazon Redshift emerges as the leading provider, closely followed by Snowflake.

Conclusion
In this blog post we explored the metadata capabilities of the leading cloud data warehouses and analyzed the information they expose through their APIs. Snowflake showcased its strength across multiple categories, excelling in tables, fields, and query metadata. Amazon Redshift demonstrated its prowess in fields and resources metadata, while Google BigQuery proved to be a strong contender in tables and observability metadata. Microsoft Synapse and Databricks showcased their expertise in user-related metadata and query performance, respectively.
But other than the quantitative competition between those giants, it’s more interesting to notice the different focus demonstrated by each vendor that sheds light on their strategies – With these cloud data applications covering a wide range of capabilities and functionalities, it’s expected that a single provider wouldn’t excel in all areas. The distribution across different categories indicates the commonalities where providers compete and the areas where they seek uniqueness. Furthermore, these different focus areas highlight the aspects that are still lacking across the board, waiting for innovation from existing players or potentially new market entrants, who potentially can change this market’s rules.