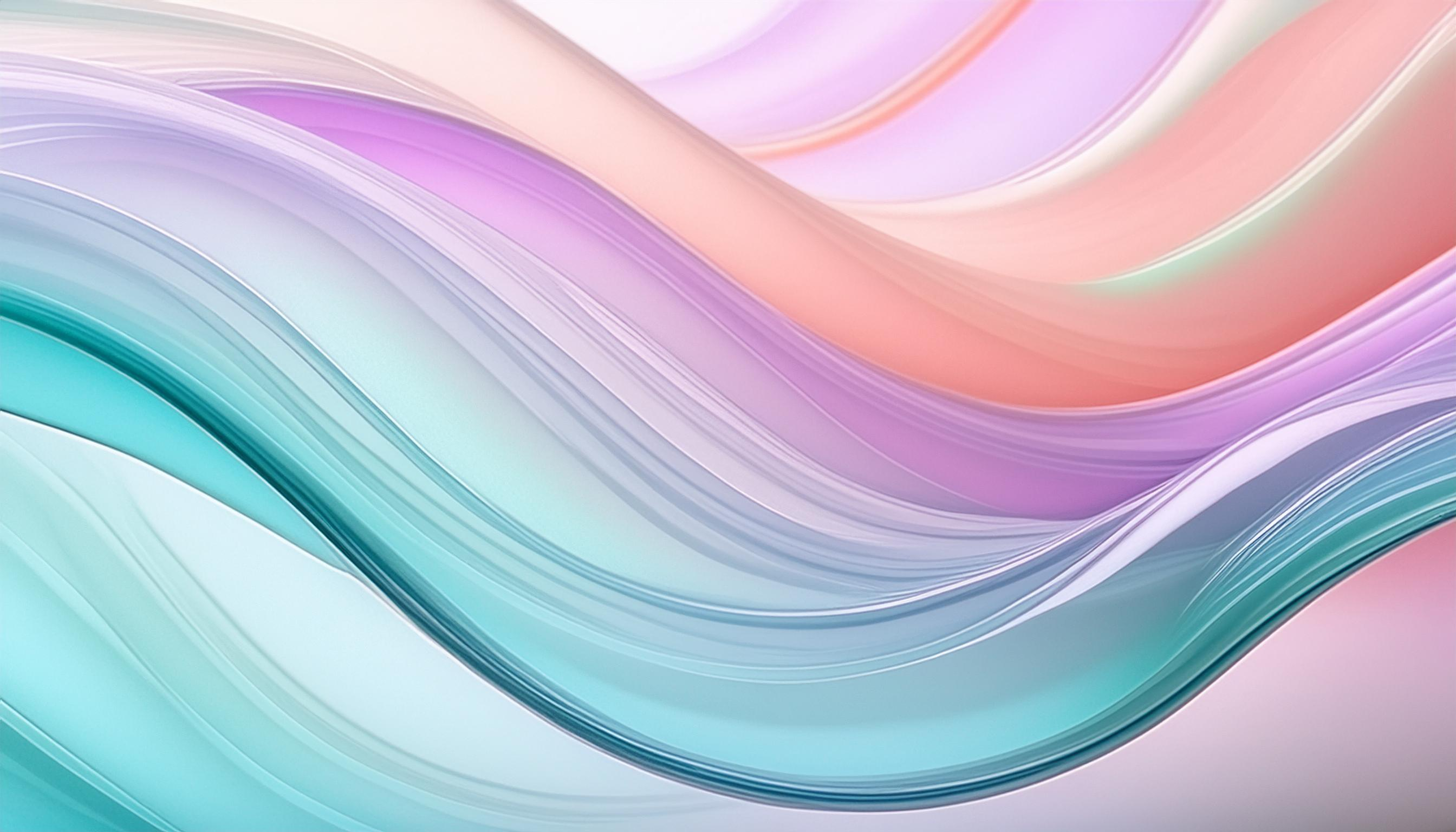
Taming the Jungles of Your Data with Semantic Data Labeling
There is a treasure trove of insights within your organizational data. More often than not, however, revealing these insights requires digging through layers of obscurity. In this vast, untamed jungle of data, semantic data labeling is your loyal machete, helping you hack away the wild weeds and undergrowth, revealing the clear path toward valuable information.
As organizations and enterprises are increasingly adopting Generative AI (GenAI) for a variety of business use cases, implementing a layer that can make data from multiple sources understandable and easy to manage becomes crucial.
What is Semantic Data Labeling?
Data labeling is assigning meaningful labels, or “tags,” to individual pieces of data within your datasets. Aimed at reflecting the actual meaning and context of the data they describe, these labels must be carefully selected and based on a formal structure called an ontology.
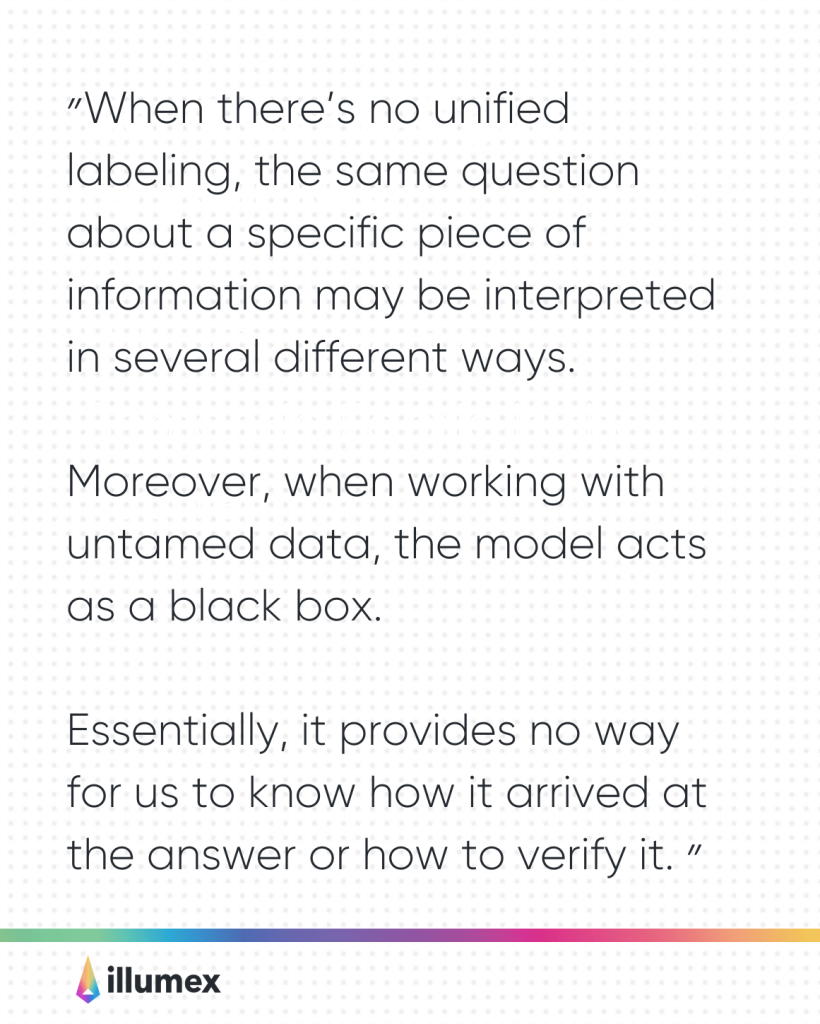
Consider ontology as a shared vocabulary, a pre-defined system of categories and relationships that govern how things are labeled. Semantic labels are, in fact, a particular type of metadata that provides data sources with annotations based on this predefined, shared vocabulary.
These labels add layers of information that help describe not only the content of the data but also its structure, the concepts it represents, and its context – its relation to other data assets. The rich contextualization and the interlinked nature of semantic labels allow machines and humans to understand and interpret data correctly. Without semantic data labeling, users encounter ambiguous, inconsistent, and poorly structured data. This hinders accurate data interpretation, reduces data processing efficiency, and complicates the integration and interoperability between different data systems.
The Benefits of Semantic Labeling
The labeling process described above brings out key abilities. Once your data speaks a common language, it becomes:
Clear: Eliminating uncertainty and errors caused by inconsistent formatting or repetitive terms. In our metaphor of a data jungle, this ensures all within an organization follow an identical route to reach a particular piece of information. With clear, meaningful definitions, time is no longer spent reconciling data, so more resources become available for insights and analysis. This, subsequently, leads to improved decision-making based on reliable conclusions and trustworthy data.
Searchable: Finding specific insights becomes instinctive as moving along familiar paths at a local park. You can use tags to organize data into specific categories based on themes, projects, operational frameworks, and analytical goals. Semantic labels let you ask questions and search using natural language, consequently simplifying the job of recognizing and investigating relevant data. Individuals within the organization are empowered to access and analyze data autonomously. The alignment with specific themes, projects, operational frameworks, and analytical objectives makes data-driven insights accessible to all.
Interoperable: Different datasets, even from various sources, can be combined and clustered together thanks to their shared semantic labels. It opens up the possibility of new connections between the routes and sections in your data jungle, eliminating silos and enabling seamless integration and analysis.
Compliant: With uniform, consistent labels and data handling procedures, data governance becomes more effective as compliance with regulations becomes easier.
Implementing personally identifiable information (PII) tagging is important for compliance and data governance. It uses predefined rules to identify and label PII in datasets, ensuring consistent application of privacy policies across the organization.
Collaborative: Tagging data entities and their downstream elements provides comprehensive coverage and relevant business and data context for various scenarios. This feature enhances organizational communication, enabling quicker response times and effective security incident management. It helps prioritize incidents, manage workloads more efficiently, and supports thorough remediation by notifying relevant stakeholders when compromised data is identified.
Unleashing the Power of GenAI
These days, when Generative AI is all the buzz, many enterprises are evaluating its potential benefits for their organization. Yet, while the benefits could be astounding, such integration is still risky. After all, GenAI models rely on the data on which they are trained. If the data within the specific domain is inconsistent, full of confusing terminology, or lacks context, the results will reflect that.
When there’s no unified labeling, the same question about a specific piece of information may be interpreted in several different ways. Moreover, when working with untamed data, the model acts as a black box. Essentially, it provides no way for us to know how it arrived at the answer or how to verify it. Consider, for instance, a lab equipment company where the Sales department defines ‘customer’ as the particular lab they sold to, while Customer Success defines the customer as the lab’s professor (who may move to a different lab or retire). How should the model answer a relatively straightforward question like “Who is our biggest customer”?
This is where semantic data labeling comes to the rescue. When the training of Large Language Models (LLMs) is based on semantically labeled data, it eliminates the need for extensive model training. Instead, the models can pick up domain expert knowledge embedded in the metadata. So that accurate semantic labeling is performed automatically and on scale.
Automated Semantic Labeling for Trustworthy Insights
Relying solely on domain experts to curate all semantics of your data is impractical. It simply does not scale. With organizational data changing every day, the semantic labeling process is ongoing; it never really ends. That’s why your semantic labeling should be augmented with a metadata-driven, automated process performed by LLMs previously trained on domain semantics. With automation handling the bulk of the work, domain experts only need to be involved for highly sensitive or conflicting definitions. As a result, generative AI systems can accurately interpret and utilize this automated yet human-curated information without requiring complex training on contextual understanding.
Well-mapped connections and clear, unified labels act as a middleman between the machine and its human users, leading to better, verifiable answers. Automated semantic labeling ensures smooth and successful integration of GenAI into your company, unlocking its full potential to generate accurate results and trustworthy insights, improve data-based decision-making across the organization, and drive innovation.
Transforming the Data Jungle Into a Mapped Landscape
In conclusion, to prepare your organization for the future and enable the smooth implementation of GenAI models, you must first clear the pathways of your data jungle and turn it into a well-mapped terrain. Automated semantic data labeling is a powerful tool that allows you to do just that.
With this automation in place, your data can speak the same language across the entire company. Silos no longer confine it, it becomes consistent and contextualized, and thanks to regular procedures and standards for labeling, compliance is now a breeze. When harnessing the abilities of GenAI, your carefully organized data forms the foundation for accurate, trustworthy results and insights.
Curious to learn more and discover how to prepare your data for advanced analytics and Generative AI?
Download our free eBook: Unlock The Power of Your Data With the Industry-Specific Semantic AI Approach.
You will learn:
- Why semantics is the new superpower for enterprises
- Industry-specific semantics and their value for data management
- How to unleash contextual institutional knowledge with LLMs and graphs
- How to maximize the Data ROI in your enterprise