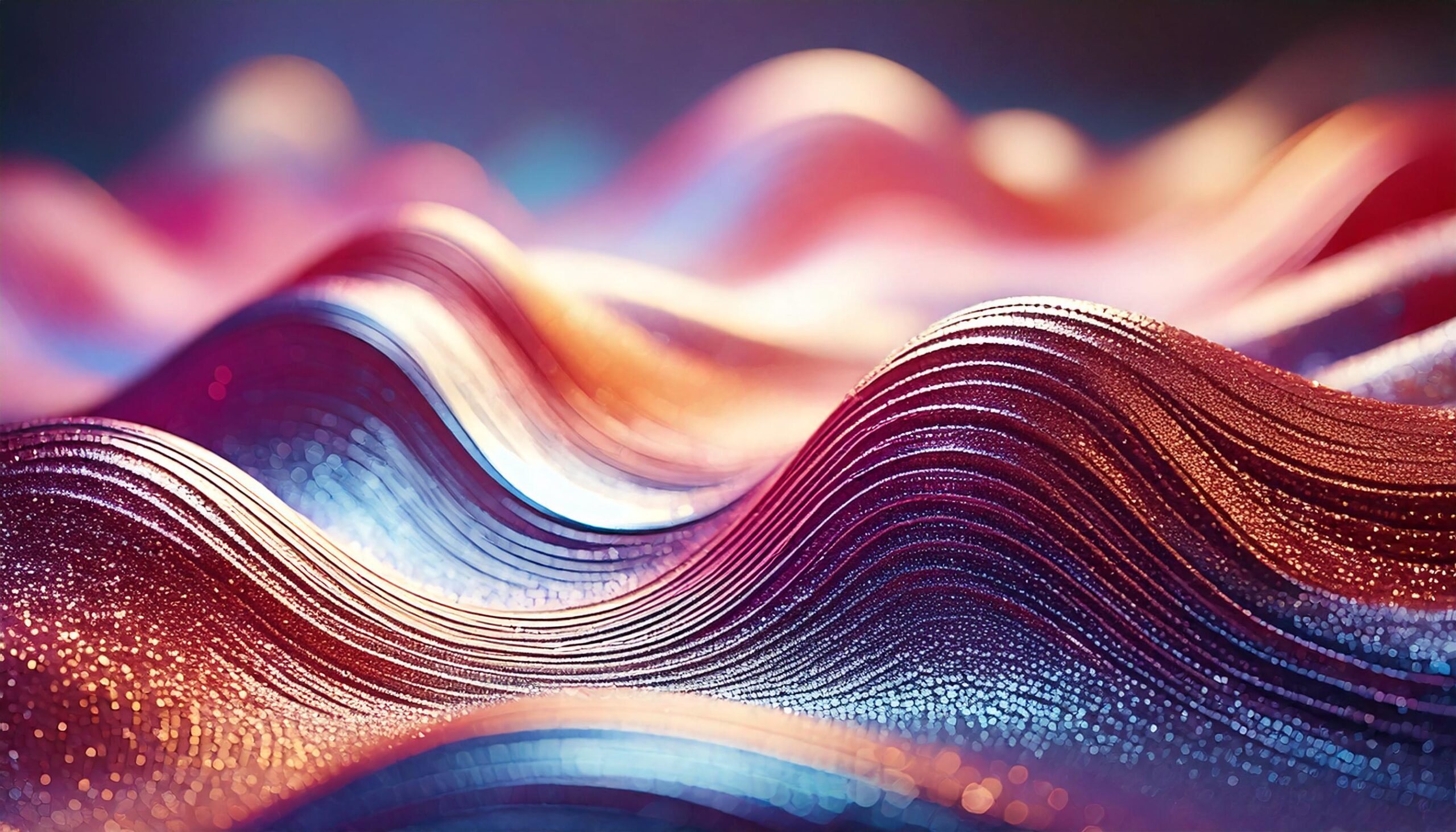
How Structured Data + GenAI Can Power Your Business Decisions
In 2024, AI adoption has skyrocketed. According to McKinsey, the adoption rate surged to 72% early this year, with Gartner noting that Generative AI (GenAI) has become the most frequently deployed AI solution across organizations. But as GenAI quickly integrates into day-to-day operations, there’s a renewed focus on what fuels its success and drives business value: data. And not just any data—AI-ready and well-governed structured data.
While the importance of GenAI deployment is clear, many companies have yet to create data strategies that truly make GenAI work for them. In fact, organizations are flooded with data but often lack the insights they need to make data-based decisions. Structured data is where the true value lies, driving revenue through more precise insights.
In this post, we’ll cover why structured data is so essential to organizational GenAI’s success and the steps you can take to make sure your data is ready for AI.
You don’t need endless manual processes to make your structured data AI-ready. illumex automates semantic alignment and context enrichment and augments governance to unlock the full value of your data.
Your insights stay precise, compliant, and business-ready with up to 90% less manual effort. Seamless AI readiness—without the complexity.
Book a demo today to see how it works.
Why GenAI Needs Structured Data
First, a quick refresher: structured data is neatly organized and easily searchable (at least, at first glance), like rows and columns in a database. Unstructured data includes files like text, audio, etc. It’s harder to analyze because it’s freeform and lacks clear categories.
Most people think unstructured data is the main challenge in deploying enterprise GenAI. And it makes sense; unstructured data makes up around 80% of all company data, so organizations are rushing to make it usable.
But structured data is where the true decision-making power lies—and it’s not as simple to make use of as the name may indicate. Structured data usually lacks the built-in context and semantics you find in unstructured data, leaving GenAI models struggling to interpret it without a lot of prep. Deploying GenAI on top of poorly prepared structured data leads to skewed insights, user distrust, and, as a result, failed projects.
And even worse, misinterpreted structured data risks inaccurate predictions, missed business opportunities, and regulatory missteps.
However, by preparing structured data for GenAI, organizations can generate precise, actionable insights. They can automate processes to scale and ultimately transform GenAI into a trusted tool for decision-making.
The Challenge of Making Structured Data AI-Ready
GenAI applications are designed to identify patterns, offer recommendations, and simplify operations. But when it comes to structured data, getting there requires more than just organizing data into tables and fields. Structured data must be labeled, contextualized, and continuously governed to truly work for GenAI. Here are some specific challenges companies face when attempting to make structured data AI-ready:
- Lack of Semantic Meaning: Structured data is typically stored in tables and columns with limited meaning and context. This makes it difficult for GenAI models to understand relationships between data entities. Without semantic meaning, usage context, and the proper industry context, GenAI can misinterpret data, leading to skewed or nonsensical outputs (yes, we’re talking AI hallucinations).
- Data Silos and Accessibility Issues: Structured data often lives in isolated systems—different departments, applications, etc.— creating silos that prevent GenAI from accessing the full data picture. This isolation limits AI’s ability to deliver reliable answers and can cause compliance issues if sensitive data is overlooked.
- Data Quality and Consistency: Inconsistent or poorly maintained structured data can also lead to inaccurate AI responses. GenAI relies on clean, high-quality data, and any inconsistencies create risks of faulty recommendations and misaligned decisions. Take duplications, for example. Using the same semantic label for several different data entities can cause confusion when looking for specific insights.
- Governance and Compliance: Structured data can contain sensitive information such as PII (Personally Identifiable Information) that must be managed for regulatory compliance. Without automated governance, companies risk non-compliance, especially in highly regulated industries where data must be carefully monitored and reported.
Each of these challenges makes structured data more difficult to work with but also more valuable. Companies that find a way to map, semantically label, reconcile, and govern their data at scale can achieve AI readiness, enabling their GenAI applications to drive meaningful, accurate business insights.
“Achieving AI readiness for structured data isn’t about doing more manual work—it’s about automating processes to scale, aligning governance with AI strategy, and adding layers of meaning and context
so that GenAI can make sense of your data.”
Structured Data is the Backbone of GenAI and Agentic Analytics Success
Structured data is the foundation of AI readiness. It allows GenAI to generate insights and make decisions that are aligned with business goals. Structured data that is enriched with meaning and context provides value through:
- Accessibility for Analysis: Data that’s accessible and easy to query is data that AI can work with. Structured data that is enriched with meaning, industry context, and the context of how the data is used within the organization, tells the right story, one that’s aligned with business objectives.
- Actionable Insights and Revenue Impact: The insights that drive strategic business decisions—and, ultimately, revenue—come from structured data. With the right structure, AI can turn raw data into meaningful insights that align with growth goals.
In short, structured data is the foundation of AI readiness. It provides GenAI with the clarity and context it needs to generate reliable insights and support informed decisions. So how do we achieve that?
Strategies for Making Structured Data AI-Ready
Getting structured data ready for AI is about creating a foundation that makes the data easily interpretable and usable by GenAI models. Here are three essential strategies to transform structured data into a valuable resource for AI-driven analytics.
- Centralized Data Consistency: Building a Single Source of Truth: A centralized data repository is like having one dependable “home base” for all relevant datasets. But consistency alone isn’t enough—adding usage and business context and semantic meaning is essential. By embedding these layers into structured data, you allow GenAI models to understand relationships and nuances between data points, creating insights that are far richer and more accurate. This setup enhances data quality and boosts its usability, ensuring AI-driven responses are precise and context-aware across a range of use cases. In short, a centralized, semantically enriched repository turns structured data from isolated points into a cohesive, actionable asset.
- Automating Metadata Management and Maintenance: Manual metadata management is time-consuming and can slow down AI-driven processes. Introducing an automated metadata management system changes the game. With this approach, metadata is continuously scanned and updated without needing hands-on adjustments. This reduces the workload on data teams, lowers maintenance costs, and minimizes the risk of human error. Automated metadata keeps your structured data environment in top shape and ready for fast, accurate analysis, ensuring AI models always work with the latest, most accurate data available.
- Enhanced Security and Governance: When it comes to your data, security and governance are non-negotiable. Strong governance measures mean that data is both accessible and safeguarded while being compliant with industry standards. This includes not only securing data sources but also managing every user interaction within the GenAI or self-serve analytics platform. Robust governance protocols guarantee data accuracy, control access based on user roles, and enforce privacy regulations, adding an essential layer of trust and accountability. This secure framework means you can confidently use AI-ready data, knowing that it’s safe, compliant, and ready to support reliable decision-making.
How illumex Prepares Structured Data for GenAI Success
Achieving AI readiness for structured data isn’t about doing more manual work—it’s about automating processes to scale, aligning governance with AI strategy, and adding layers of meaning and context so that GenAI can make sense of your data. Here’s how illumex can help transform structured data into a reliable foundation for GenAI success:
1. Automated Semantic Labeling and Context Enrichment
Automatically adding semantic labels to structured data allows GenAI to interpret data points more accurately. illumex automatically maps, labels, and enriches your metadata with semantic, business, and industry context, creating a virtual knowledge graph (your Generative Semantic Fabric—GSF). Providing GenAI models with essential context helps the model “understand” relationships between data points.
2. Breaking Down Data Silos for Full Accessibility
Siloed data creates a disjointed view of information, preventing GenAI from accessing all the data needed to produce accurate insights. illumex’s Generative Semantic Fabric allows organizations to unify data across departments through active metadata management. This makes room for a seamless flow of structured data for GenAI—without ever needing to physically move data from its sources. By creating a centralized source of truth, companies can provide GenAI with complete, reliable data that reflects every aspect of their operations. This not only improves output accuracy but also helps companies remain compliant with regulatory requirements.
3. Continuous Data Quality Monitoring and Maintenance
Data quality isn’t static; it requires constant monitoring to allow accuracy and consistency. illumex provides organizations with tools to automatically reconcile and suggest proper naming conventions and definitions, while continuously flagging inconsistencies, and performing corrections where needed.
Whenever data or semantic entities change, illumex notifies the assigned owner with all the details. It shows exactly what changed and how it affected other entities. The platform also highlights the specific asset’s autogenerated column-level lineage, so there’s a clear view of the impacted downstream. This proactive approach to data quality means that structured data remains accurate, supporting AI models with reliable information. When structured data is high-quality and consistent, companies can trust their GenAI insights, making better, faster decisions.
4. Scalable Governance to Meet Compliance and Security Needs
Compliance and security are essential for any enterprise AI deployment. illumex augments data governance, automating repetitive tasks, and ensuring that structured data meets regulatory requirements without needing continuous manual intervention. With automated documentation, rule-based checks, and more, companies gain peace of mind knowing that sensitive data is managed responsibly and in accordance with industry standards.
With structured data that’s both secure and compliant, organizations can scale GenAI across their operations confidently, knowing they’re safeguarded against regulatory risks.
Making Structured Data AI-Ready for Better Decisions
Structured data is where true business value lies—both in terms of decision-making and revenue impact. But to unlock its potential, structured data must be AI-ready: it needs to be well mapped, enhanced with semantic meaning and business (and usage) context. It also requires ongoing maintenance, and continuous governance, because AI-readiness must evolve along with your growing data estate.
With illumex’s approach to automated mapping, labeling and reconciliation, centralized data access, continuous quality monitoring, and scalable governance, companies can transform structured data into a foundation of reliable, actionable insights. Structured data is more than a technicality; it’s the difference between AI that delivers reliable self-serve analytics and AI that erodes trust.
Getting structured data AI-Ready is a smart investment for businesses looking to unlock GenAI’s full potential. Investing in automated, scalable processes for AI readiness will result in aligned data with business goals—driving insights that matter.